Back to Journals » International Journal of General Medicine » Volume 16
Hematological, Inflammatory, Coagulation, and Oxidative/Antioxidant Biomarkers as Predictors for Severity and Mortality in COVID-19: A Prospective Cohort-Study
Authors Smail SW , Babaei E, Amin K
Received 23 December 2022
Accepted for publication 10 February 2023
Published 17 February 2023 Volume 2023:16 Pages 565—580
DOI https://doi.org/10.2147/IJGM.S402206
Checked for plagiarism Yes
Review by Single anonymous peer review
Peer reviewer comments 2
Editor who approved publication: Dr Scott Fraser
Shukur Wasman Smail,1 Esmaeil Babaei,2,3 Kawa Amin4
1Department of Biology, College of Science, Salahaddin University, Erbil, Iraq; 2Department of Biology, School of Natural Sciences, University of Tabriz, Tabriz, Iran; 3Department of Pharmacognosy, College of Pharmacy, Hawler Medical University, Erbil, Kurdistan Region, Iraq; 4College of Medicine, University of Sulaimani, Sulaymaniyah, Iraq
Correspondence: Shukur Wasman Smail, Salahaddin University, Erbil, Iraq, Tel +9647504491092, Email [email protected]
Purpose: Oxidative stress (OS) and inflammation are pivotal points in the pathophysiology of coronavirus disease-2019 (COVID-19). This study aims to use routine laboratory and oxidative stress/antioxidative biomarkers as predictors for the mortality of the disease.
Patients and Methods: This prospective cohort study, made up of 120 COVID-19 patients from emergency units in Erbil, Duhok, Kirkuk, and Sulaymaniyah cities in Iraq, from May the 1st to May the 30th, 2021, and 60 healthy controls (HCs) (n = 60). The patients were re-categorized into mild (n = 54), severe (n = 40), and critical (n = 26) groups based on the clinical criteria. Following admission to the hospital, blood was directly collected for measuring routine laboratory biomarkers.
Results: Neutrophils and neutrophil/lymphocyte ratio (NLR) were higher in the critical group, while lymphocytes were lower in the severe and critical groups compared to the mild group. The CRP, ferritin, and D-dimer values were more elevated in severe and critical cases than in mild COVID-19 cases. The levels of malondialdehyde (MDA), nitric oxide (NO), and copper were elevated, while the superoxide dismutase (SOD) activity level and total antioxidant capacity (TAC) level were lower. However, vitamin C, glutathione peroxidase (GPx), and catalase activity levels were not changed in the COVID-19 groups compared to the HCs. NO and ferritin were predictors of ICU hospitalization; D-dimer, MDA, and NLR were predictors of mortality. NO, and NLR were predictors of SpO2 depression. Moreover, NO, and copper have both good diagnostic values, their cutoffs were 39.01 and 11.93, respectively.
Conclusion: There is an association between immune dysregulation and oxidative imbalance. The biomarkers, that could be considered as predictors for the severity and mortality of COVID-19, are the NLR, NO, ferritin, and D-dimer. The age equal to and older than 50 has a poor prognosis in the Kurdish population.
Keywords: COVID-19, inflammation, mortality, oxidative stress, predictors
Graphical Abstract:
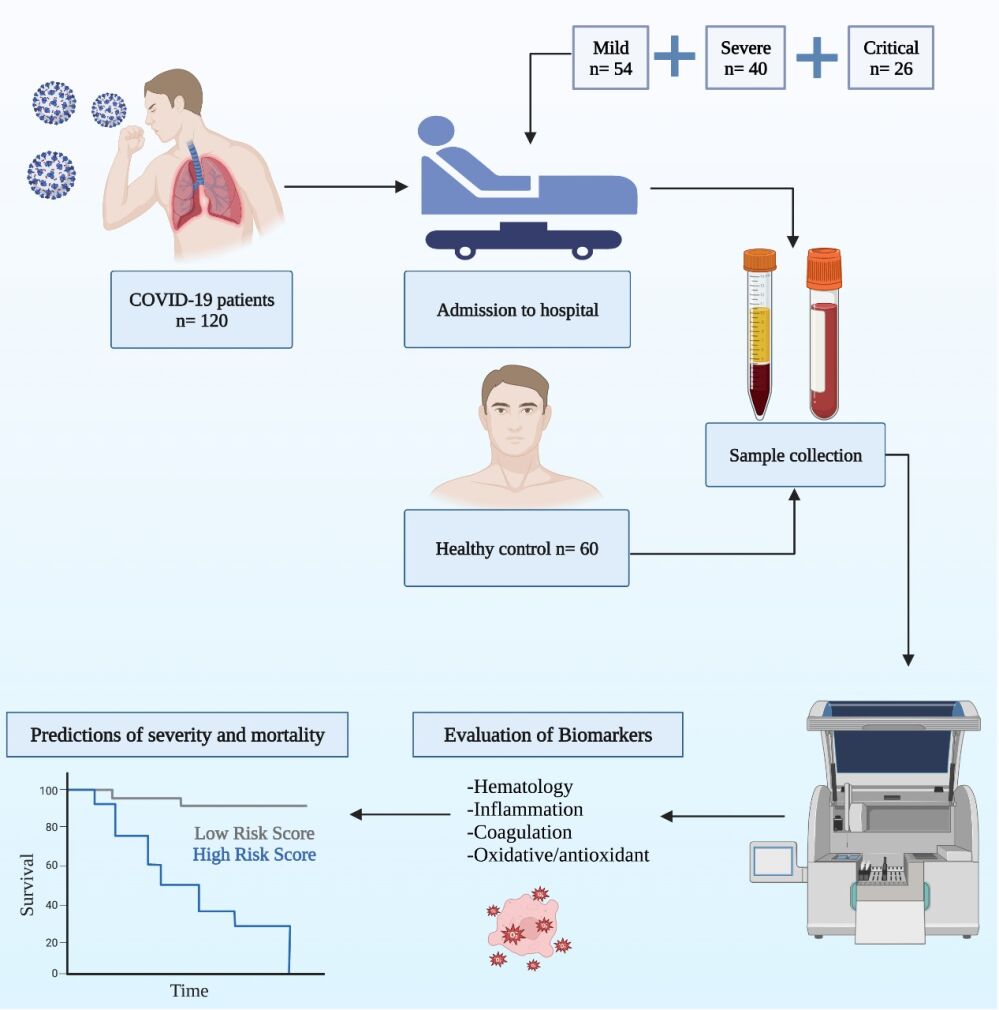
Introduction
In December 2019 in the Wuhan region of China, several patients were reported with respiratory alterations because of the new beta coronavirus, known as severe acute respiratory syndrome-coronavirus-2 (SARS-CoV-2).1 This highly contagious virus has already spread to a growing number of countries, and this new disease coronavirus disease-2019 (COVID-19) was declared a pandemic by the World Health Organization on March 11, 2020.2 The disease could be asymptomatic or generate mild, severe, or critical symptoms.3
Clinical laboratory parameters have been a fundamental part of classifying the severity and prognosis of COVID-19 including very high levels of D-dimer, ferritin, and C-reactive protein (CRP) along with the decreased systolic blood pressure and oxygen saturation (SpO2).4 Therefore, the consultants suggest intense monitoring of SpO2 in COVID-19 patients and when it falls below 94%, the supplementation of oxygen is recommended.5 Therefore, blood gas values are promising biomarkers for COVID-19 diagnosis and prognosis.6
A key stage in the diagnosis and prognosis of COVID-19 is the examination of routine laboratory biomarkers.7–9 Furthermore, alterations in blood values such as neutrophilia, lymphopenia, and high neutrophil/lymphocyte ratio (NLR) were also common among COVID-19 patients.10 Hence, the neutrophil-to-lymphocyte ratio (NLR) is utilized as an inflammatory biomarker.11
Factors such as age and sex have been recognized as the primary predictors of COVID-19 severity and mortality.12,13 Likewise, comorbidities such as obesity, epilepsy, dementia, diabetes mellitus (DM), and hypertension (HTN) are associated with COVID-19 mortality.14,15 In the severe form of COVID-19, the immune system cells produce more proinflammatory cytokines, which results in a cytokine storm (CS). The latter is linked to a redox metabolism imbalance, leading to increased oxidative molecular damage, reduced antioxidant bioavailability, modulation of signal transduction pathways, and alteration of gene expression. Consequently, the accelerated progression of systemic inflammatory response and multi-organ failure.16
It is known that the oxidant-antioxidant balance is an important mechanism for homeostasis in an organism. Oxidative stress (OS) is characterized by an increase in the level of oxidants and/or a decrease in antioxidant capacity and is postulated to occur when an imbalance shifts towards oxidants.17 One of the pathophysiologies of SARS-CoV-2 infection is thought to be an imbalance of OS and antioxidant status.18
The OS effects of COVID-19 can be revealed by measuring OS biomarkers with antioxidants. One of the vital OS biomarkers is malondialdehyde (MDA), a metabolite that emerges from lipid peroxidation, and Nitric oxide (NO), which is a product of free radicals and that could act as a vasodilator.19,20 The damaging effects of OS can be reversed by antioxidants. There are two groups of antioxidants: enzymatic and non-enzymatic. The enzymatic antioxidants include superoxide dismutase (SOD) that scavenges superoxide anion (O2−) levels, and glutathione peroxidase (GPx) which catalyzes the reduction of H2O2 or lipoperoxide (L-OOH) to water and alcohol, respectively.21 Catalase, like GPx, is responsible for eliminating H2O2 but it acts in the presence of high concentrations of H2O2.22,23 However, the non-enzymatic antioxidants include vitamin C, and metallic ions (copper and zinc). Copper and zinc incorporate into SOD-1, while vitamin C protects the lipid membranes from peroxidation, through the regeneration of the active form of vitamin E.24
Several studies have stated the high level of OS and deficient antioxidant biomarkers in COVID-19 patients.25–27 However, these investigations do not provide adequate justification for the use of antioxidant parameters as diagnostic and prognostic biomarkers. Therefore, the goals of the current study are to determine the impact of OS on COVID-19 sufferers through the measurement of hematological, inflammatory, coagulation, and oxidative/antioxidant biomarkers. The utilization of these biomarkers as predictors for diagnosis, prognosis, and their association with severity and mortality are also under the scope of this study.
Materials and Methods
Patients
This multicenter prospective cohort study was carried out from May the 1st until May the 30th, 2021. There are 180 participants included in this study categorized into 60 healthy controls (HCs) (26 males and 34 females) and 120 COVID-19 patients (60 males and 60 females), as it’s shown in Table 1. The latter, who attended the hospital’s emergency departments in Erbil, Duhok, Kirkuk, and Sulaymaniyah in Iraq, had symptoms compatible with a SARS-CoV-2 infection. A swab of the nose and mouth was taken and the results were confirmed by RT-PCR, which detected SARS-CoV-2. Moreover, COVID-19 participants were distributed into three subgroups, including mild group (n = 54), severe group (n = 40), and critical group (n = 26) following Chinese regulations with minor alteration.28,29 However, the Chinese regulation protocol divided COVID-19 patients into mild, moderate, severe, and critical groups, while the current study combined mild and moderate groups into mild group. According to Chinese regulation protocol, mild cases either do not exhibit pneumonia or exhibit mild pneumonia; severe cases manifest pneumonia characterized by shortness of breath and their SpO2< 93 and critical patients suffer from respiratory failure, septic shock, or multiple organ failure.29 All patients in the critical group died in the hospital during the thirty-day follow-up. Furthermore, all COVID-19 patients were non-vaccinated against COVID-19 disease, and the patients were not involved in any therapeutic intervention. The exclusion criteria for patients included a lack of access to clinical information and the absence of any laboratory parameters included in this study. Patients who used drugs before being admitted to the hospital were also excluded.
![]() |
Table 1 Clinical and Demographic Characteristics of Healthy Control and COVID-19 Patients |
Sample and Data Collection
All participants underwent a blood sample collection at the time of admission before taking any drugs. The phlebotomy was performed, and a ten-milliliters syringe was used for drawing venous blood and then placing it in serum separator tube (SST), sodium citrate, and ethylenediaminetetraacetic (EDTA) tubes. Following centrifugation of the SST and sodium citrate tubes at 3000 rpm for 15 minutes to separate the serum and plasma, an aliquot was taken and placed into Eppendorf tubes to be kept at −20 °C until further analysis. The blood in the EDTA tube was immediately utilized for measuring the complete blood count. Demographic data, medical history, and comorbidities were obtained from the hospital’s data management system. All participants were carefully monitored from the time they were admitted until the end of the study. The follow-up was scheduled at regular times, and survival time in this study was the duration starting from the time of admission to the time of death (for non-survivors) or the time of discharge (for survivors).
Methods for Measurement of Parameters
Measurement of Routine Laboratory Parameters
The laboratory parameters were measured as follows; D-dimer and CRP were determined by Cobas c311 (Roche, Germany), while Ferritin measurement was achieved by COBAS e411 (Roche, Germany). Additionally, lymphocytes and neutrophils were measured by Beckman Coulter and SpO2 was measured by a Masimo pulse oximeter.
Measurement of Oxidative Stress Biomarkers
Measurement of serum MDA was done by spectrometric calculations.30 The principle of MDA is based on the formation of lipid peroxidation; first, it reacts with thiobarbituric acid (TBA) to form a measurable MDA-TBA compound. In the test tube, a working solution was prepared by adding 150 µL of serum with 1 mL of 17.5% trichloroacetic acid (TCA), then 1 mL of 0.66% TBA was added to the working solution. After mixing and vortexing for 1–2 minutes, the samples were put into a water bath and boiled at 95 ◦C for 45 minutes. Thereafter, the samples were cooled at room temperature and consequently,1mL of 70% TCA was added to the mixture. After that, it was centrifuged for 15 minutes at 3000 rpm. The pink-colored supernatant was then read at 532 nm by the spectrometer and the absorbance was converted into concentration.
To determine serum NO concentration, the Griess reaction was used, which was first reported by Griess.31 The half-life of NO is very short; therefore, the NO level was difficult to measure, hence it was converted to nitrite NO2 and nitrate (NO3); the measurement of total NO2 and NO3 concentration was the reflection of NO concentrations in this study. According to the manufacturer procedure, salts of transient diazonium in an acidic medium will be formed after treatment of NO2 with a diazotizing reagent, eg, sulfanilamide. To form a stable azo compound, this salt undergoes a reaction with the coupling reagent N-naphthyl-ethylenediamine (NNED). An intense purple color was formed, and its absorbance was measured at 540 nm, which directly corresponds to NO3 concentration.32
Measurement of Antioxidative Stress Biomarkers
Determination of serum SOD activity was performed through the concept given by the scientists In which quercetin undergoes oxidation by O2.33,34 Standard solutions were prepared by adding 0.8 mM tetramethylethylenediamine (TMEDA) and 0.08 mM EDTA together in a clean tube of buffer solution (pH = 9) containing 16 mM potassium sulfate. Then, 100 μL of serum and 20 μL of quercetin were added together, and after 20 minutes, the optical density was measured by a spectrophotometer at 406 nm.
Trace minerals (zinc and copper) were measured colorimetrically according to the manufacturer’s instructions (Randox, UK). The measurements of Vitamin C, catalase activity, GPx activity, and TAC were achieved after following the steps of the manufacturer (Elabscience, USA).
Statistical Analysis
The SPSS 28 (IBM, USA), GraphPad Prism 9 (GraphPad Software, Inc., USA), and MedCalc 20 (MedCalc Software, Ltd., Belgium) were used for statistical analysis and demonstrating the graphs. The data did not pass the normality tests (Shapiro–Wilk and D’Agostino). For comparison and correlation between groups, Kruskal–Wallis and Spearman tests were utilized, respectively. Dunn’s test was applied as a post-hoc test for multiple comparisons. For the prediction of the effect of parameters on ferritin, CRP, D-dimer, and SpO2, multivariate regression was applied. COVID-19 mortality and intensive care unit (ICU) hospitalization were studied using binary multivariate logistic regression. Regression coefficient (B) and odds ratio (OR) were used to know the degree of association of biomarkers to mortality in the regression model. The diagnostic significance of laboratory parameters was determined using cut-off in the ROC curve; the area under curve (AUC) of each biomarker was used to discriminate HC from COVID-19. The predictive accuracy of biomarkers was tested by positive predictive value (PPV) and negative predictive value (NPV).
The hazard ratio (HR) was calculated using Cox proportional-hazards regression to determine the association of variables with mortality in COVID-19 while Kaplan-Meier was utilized for survival analysis. *p-value < 0.05,** p-value < 0.01, *** p-value < 0.001 and **** p-value < 0.0001.
Results
Demographic Characteristics, Biochemical, and Hematological Biomarkers
Sixty HCs and 120 COVID-19 patients enrolled in this study. 43.33% of HCs were males and 56.67% of them were females. Regarding COVID-19 patients, 50% of them were males and 50% of them were females. The median ages of HCs and COVID-19 are 37.00 (26.00–47.00) and 47.50 (35.50–55.00), respectively. In addition, COVID-19 participants were further sub-grouped into the “mild group” 54 (45%), “severe group” 40 (33.33%), and “critical group” 26 (21.67%). The most frequent associated comorbidities were: HTN (42.85%), multiple comorbidities (23.81%), DM (19.05%), cancer (4.76%), and other diseases (9.53%) (Table 1).
A significant difference (p-value<0.0001) was observed in the median age of severe and critical groups of COVID-19 patients compared to HCs; in addition, a significant difference was also observed in the median age of critical groups of COVID-19 patients compared to the median of mild groups (Table 2, Figure 1a).
![]() |
Table 2 Comparison of Different Parameters Among Healthy Controls and Different Groups of COVID-19 Patients |
With a p-value of 0.0001, there were significant differences in the median of CRP in the severe and critical groups compared to the HCs and mild groups (Table 2 and Figure 1b). Meanwhile, neutrophil and NLR were significantly higher in patients with severe and critical COVID-19 compared to mild patients (p-value<0.0001) (Table 2, Figures 2a and c). Compared to the mild group, lymphocytes were lower in the severe and critical groups significantly (p-value<0.0001) (Table 2 and Figure 2b). As shown in Figures 2d and e, ferritin and D-dimer were significantly higher in patients with severe and critical COVID-19 compared to mild patients (p-value<0.0001). In addition, SpO2 presented a marked decrease in the severe and critical groups when compared to the mild group (p-value< 0.0001), but it did not show significant differences between the severe and critical groups (Table 2 and Figure 2f).
Evaluating Oxidative Stress Biomarkers Among Studied Groups
COVID-19 patients who were in critical groups had higher median concentrations of MDA than HCs, mild, and severe groups (p<0.0001) (Table 2 and Figure 3a). Non-significant differences in the median of NO were found between HCs and mild, severe, and critical groups; by contrast, significant differences were found for NO levels in the serum among other groups (p<0.0001) (Table 2 and Figure 3b).
Comparing Anti-Oxidative Biomarkers Among Studied Groups
Significant differences (p<0.001) were observed in terms of trace elements (zinc and copper) in serum among the studied groups. As found in Table 2 and Figure 4a, significant differences (p<0.001) were observed in the median of zinc, which was significantly lower in the group of COVID-19 cases with respect to the control group (82.71 (67.78–104.5)). As can be seen in Table 2 and Figure 4b, serum levels of copper were significantly higher in mild (10.91 (3.409–17.40)), severe (15.83 (12.27–19.41)), and critical (18.90 (12.15–27.15)) groups compared to HCs (8.012 (1.321–10.06)). Table 2 and Figure 4c showed that there were no significant differences (p-value = 0.916) in the amount of vitamin C between the groups that were studied (Table 2, Figure 4c).
Anti-Oxidative Enzyme Activity and TAC Among Studied Groups
Serum levels of SOD activity were significantly lower (p-value = 0.0007) in the COVID-19 patients who presented severe and critical complications compared to HCs. Whereas non-significant differences were observed for GPx (p-value = 0.097) and catalase (p-value = 0.203) activity among the studied groups (Table 2, Figure 5). Regarding TAC, a significant difference (p-value = 0.030) was only found between the severe group and HCs (Table 2 and Figure 5d).
Constructing Binary Multivariate Logistic Regression Model for Predicting ICU Hospitalization and Death of COVID-19 Patients
The construction of the models was carried out using binary multivariate logistic regression. To select the variables that made up the model, the “forward conditional” method was used. Model calibration was evaluated through the Hosmer-Lemeshow goodness-of-fit test. A significance of 0.05 was taken as a reference to decide on its usefulness (if p>0.05, the model is adequate), which means that there are no significant differences between the observed results and those predicted by the model.
Regarding the prediction of the association of the independent variables (lymphocyte, neutrophil, ferritin, NLR, SOD, zinc, copper, CRP, D-dimer, GPX, catalase, NO, MDA, TAC, and vitamin C) with ICU hospitalization, statistically significant associations were observed for NO (p<0.0001, OR = 1.241) in model 1, for ferritin (p = 0.016, OR = 1.010) and NO (p = 0.007, OR = 1.159) in model 2. The other variables did not improve the explanatory power of regression, so they were excluded from the regression model (Table 3).
![]() |
Table 3 Binary Multivariate Logistic Regression Analysis for ICU Hospitalization and Death |
According to the data in Table 3, the explanatory variables (lymphocytes, neutrophils, ferritin, NLR, SOD, zinc, copper, CRP, D-dimer, GPX, catalase, NO, MDA, TAC, and vitamin C) were applied to know the association of them with the deaths of COVID-19 patients. Three models were constructed and tended to be associated with death in COVID-19 patients: model 1 includes D-dimer (p<0.0001, OR = 1.104), model 2 includes D-dimer (p = 0.04, OR = 1.132), and MDA (p = 0.01, OR = 1.365) and model 3 includes D-dimer (p = 0.296, OR = 1.133), MDA (p = 0.481, OR = 3.356), and NLR (p = 0.497, OR = 3.196). The other variables did not improve the explanatory power of regression, so they were excluded from the regression model. Even though D-dimer has a significant association with mortality in models 1 and 2, it is a poor predictor of mortality because its OR is slightly greater than one.
Multivariate Regression Analysis for CRP, Ferritin, D-Dimer, and SpO2
Multivariate regression was used to build the models, and the “stepwise” method was utilized to choose the variables that went into the models and acted as predictors. The calibration of the model was checked by looking for if the related variables had multicollinearity (tolerance and variance inflation factor (VIF)).
The independent variables (lymphocyte, neutrophil, NLR, SOD, zinc, copper, D-dimer, GPx, catalase, NO, MDA, TAC, and Vitamin C) were used to determine their relationship with the dependent variables including ferritin, CRP, D-dimer, and SpO2. As seen in Table 4, neutrophil, NLR, and NO act as predictors for increasing CRP (B=7.814, P<0.0001), ferritin (B=23.476, P<0.0001), and D-dimer (B=0.629, P<0.0001), respectively. NLR (B = −2.543, P<0.0001) in model 1 and NLR (B = −1.928, P<0.0001) and NO (B = −0.229, P=0.040) in model 2 act as predictors for depression of SpO2 in COVID-19 patients. The variables that did not improve the model were excluded from the regression equation. In model 1, the regression coefficient for NLR for SpO2 prediction is −2.543. (Table 3). This means that for every unit increases in NLR, there is a 2.543 folds decrease in SpO2.
![]() |
Table 4 Multivariate Regression Analysis to Predict the Effect of Independent Variables on CRP, Ferritin, D-Dimer, or SpO2 |
Correlation Analysis Among Variables
The Spearman correlation was done between biomarkers (lymphocytes, neutrophils, NLR, SOD, zinc, copper, GPx, catalase, NO, MDA, TAC, vitamin C, D-dimer, CRP, and ferritin). We found that NLR and NO were significantly associated with CRP, ferritin, and D-dimer. The detailed correlations can be seen in Table 5.
![]() |
Table 5 Correlation Analysis Among Independent Biomarkers |
ROC Curve Analysis for Diagnosis of the Disease
In Table 6 and Figure 6, ROC curves were constructed for the diagnosis of COVID-19 patients. Area under curve (AUC) has been reported for the diagnosis of COVID-19, which is equal to 0.903, 0.517, 0.807, 0.795, 0.827, 0.528, 0.680, 0.740, 0.579, and 0.570 for CRP, MDA, NO, zinc, copper, vitamin C, TAC, SOD, GPx and catalase, respectively.
![]() |
Table 6 ROC Curve to Assess Diagnostic Value of Biomarkers in Patients with SARS-CoV-2 |
The results also showed that the AUCs with the best performances in biomarkers were CRP, copper, and NO. They allow us to predict the diagnosis of the disease with a sensitivity of 83.33%, 66.67%, and 70% and a specificity of 83.33%, 96.67%, and 86.67%, respectively. The cut-off values for the CRP, copper and NO levels in the serum for diagnosis are 0.95, 39.01, and 11.93, respectively. The details of the ROC curve characteristics for other biomarkers are shown in Table 6 and Figure 6.
Kaplan-Meier Survival and Cox-Regression Analysis for Prediction Effect of Age, Comorbidities, and Gender on Mortality
The result was of survival analysis plotted on the Kaplan-Meier curve presented in Figure 7. Univariate cox-regression analyses showed that age equal to or older than fifty was significantly associated with mortality (HR = 15.90, p-value = 0.0002), while gender (HR = 0.539, p-value = 0.154) and comorbidities (HR = 1.378, p-value = 0.432) were not (Table 7).
![]() |
Table 7 Univariate Cox-Regression Analysis of Age, Gender and Comorbidities for Predicting Mortality in COVID-19 |
Discussion
The excessive response of the body’s immune system after encountering SARS-CoV-2 generates hyperinflammation distinguished by elevated levels of free radicals and CS, which results in lung damage.35 There are several biomarkers and models available that could be used for diagnosis, prognosis, and monitoring of COVID-19 patients, yet few are utilizing oxidative and antioxidative biomarkers to diagnose and predict outcomes in COVID-19 patients.36–40
In the current study, 50 years or older COVID-19 patients were significantly associated with increased mortality. The reason for this could be due to the presence of comorbidities in older age.41 Studies have shown that COVID-19 patients with lymphopenia status have dysregulation of the immune response, which is responsible for the exaggeration of a systemic inflammatory response that will be harmful to the host.42,43 This is in line with our study that showed lymphopenia was the most common symptom in the severe patient group. Lymphopenia may occur due to the migration of lymphocytes from the circulation to the lung44,45 or depletion of T-lymphocytes due to the expression of inhibitor molecules on their surfaces.46
Our data illustrates that NLR is a predictor of SpO2 depression, ICU hospitalization, and mortality of the disease. These findings are similar to Vafadar and his colleagues that demonstrated NRL association with mortality in COVID-19,47 while Yang et al11 displayed that NLR was a poor prognostic factor associated with unsatisfactory outcomes in COVID-19 patients. Therefore NLR is considered a reliable, sensitive biomarker of inflammation that interacts between the innate immune response (neutrophils) and the adaptive immune response.48
One of the common symptoms of COVID-19 is hyperinflammation which can be evaluated through laboratory parameters such as CRP, ferritin, and D-dimer. The results of this study showed an elevated level of hyperinflammation parameters in severe and critical COVID-19. Our finding is in agreement with the results of Farid et al49 who reported the elevated level of these parameters in pneumonia rather than in asymptomatic COVID-19 patients. Recently it has been claimed that CRP is the excellent diagnostic of inflammatory biomarkers in children with COVID-19 that suffered from the multisystem inflammatory syndrome.50 Another study found an association between an elevated level of D-dimer, which is considered a coagulation biomarker and an increased mortality rate in COVID-19. Soni et al51 and our data support these claims.
The current study considered ferritin as a predictor of ICU admission. This result was in concordance with the findings of Shakaroun et al.52 Oxidative imbalance in COVID-19 patients can be determined by measuring oxidative stress biomarkers such as MDA and antioxidant biomarkers. Our study shows a higher level of MDA in the critical group than in HCs, this is consonance with the published work by Avila-Nava et al,25 Mehri et al.53 The reason for increasing MDA may be due to high levels of free radicals in COVID-19 that accelerate the rate of lipid peroxidation.
In the current study, the level of NO was higher in COVID-19 patients than in control subjects. However, this is not in concordance with the study of Dominic et al.54 The role of NO depends on its concentration and site of production.55 NO in small concentrations has vasodilator and antiviral properties56 while in high concentrations, acts as a precursor of free radicals.57 Our study shows that high NO levels were associated with ICU hospitalization, depression of SpO2, and high levels of CRP, D-dimer, and ferritin. Consequently, according to the results of the ROC curve, NO levels could be used as a biomarker for the diagnosis of COVID-19.
Whether an imbalance in the trace minerals (copper and zinc) cause OS in SARS-CoV-2 patients remains debatable In this study, zinc levels were lower in groups of COVID-19 patients compared to HCs. It has also been claimed that zinc is responsible for lymphocyte development,58 so its deficiency is associated with a decrease in lymphocyte count in COVID-19, which is in line with our study. Zinc acts as an antioxidant mineral through incorporation into antioxidant enzymes, including SOD-1,59 so the deficiency of zinc makes the OS overwhelm the antioxidation system.
Surprisingly, a higher level of copper was found among COVID-19 in this study. This finding is contrary to the published word by Muhammad et al60 who reported a copper deficiency in COVID-19 patients. Copper acts as an antioxidant agent through regulation of SOD-1 activity, and it’s found that high copper levels in zinc-deficient SOD-1 play a critical role in driving OS by increasing tyrosine nitration in proteins.61 Zinc-deficient SOD-1 has exposed copper that dysregulates the oxidative/antioxidant state through NO and peroxynitrite production.62
This study also examines the levels of antioxidant enzyme activity (SOD, GPx, and catalase), antioxidant vitamin (vitamin C), and other antioxidant biomarkers (TAC). Accordingly, the level of SOD was lower in severe and critical COVID-19 patients, this finding was similar to Muhammad et al60 and Yaghoubi et al63 who found a decreased level of SOD in COVID-19 patients. It is possible that the lowered SOD is caused by the deficiency of zinc, a vital element for SOD-1, or it could be that the OS has become too intense for SOD to cope with.
Our data show decreased serum TAC levels in COVID-19 patients compared to healthy participants. This result complies with the finding by Yaghoubi et al.63 The depression of TAC is caused by the persistent OS triggering by SARS-CoV-2, making the antioxidant system overwhelmed or due to decreased levels of zinc and SOD, as confirmed by the current study. Vitamin C levels did not change in COVID-19 patients. This finding was contrary to most papers done on COVID-19, which reported that its level was deficient.64,65 It may be that the Kurdish people’s efficient intake of vitamin C-rich fruits keeps their vitamin C levels from being deficient during COVID-19.
One of the limitations of this study is the restricted number of analyzed samples used in this study, which limits the evaluation of the results. Eventually, the data used in this investigation dated back to more than a year ago, when different SARS-CoV-2 variants were prevalent worldwide, including in Iraq.66 However, the omega SARS-CoV-2 forms are currently the most common worldwide.67 Despite evidence of the existence of an alteration of redox balance in COVID-19, it is still required to carry out cohort studies with a larger sample size that explain the mechanism by which the SARS-CoV-2 produces this wide range of such alterations, as well as their exact prevalence, to find effective strategies for prevention, diagnosis, treatment, and rehabilitation of these conditions.
Conclusion
In conclusion, our findings suggest that the oxidative and inflammatory damage induced by SARS-CoV-2 may be a consequence of an imbalance between ROS production and antioxidant defenses. This study highlighted the significance of NO, D-dimer, ferritin, and NLR measurements in predicting severity and mortality in COVID-19 patients. Measuring CRP, NO, and copper levels in COVID-19 patients upon hospital admission has been shown to have considerable diagnostic importance. Our cohort study implies that age could be a predictor of COVID-19 patient mortality.
Data Sharing Statement
The data used and/or analyzed during the current study are available from the corresponding author upon reasonable request.
Ethics Approval and Consent to Participate
This study was authorized by Salahaddin University-(SUE) Erbil’s ethics committee following the Helsinki Declaration (approval number: R12-021; 96 approved on April 18, 2021). All participants gave their written informed consent before blood withdrawal. For patients who were unable to sign due to life-threatening emergencies, we asked his or her relatives, who had substitute decision-makers, legal guardians, or power of attorney for the patient.
Consent to Publish
The authors affirm that all participants provided informed consent for publication.
Acknowledgments
The authors wish to thank the University of Tabriz for logistical support.
Author Contributions
All authors made a significant contribution to the work reported, whether that was in the conception, study design, execution, acquisition of data, analysis, and interpretation, or in all these areas; they took part in drafting, revising, or critically reviewing the article; they gave final approval of the version to be published; they agreed on the journal to which the article had been submitted; and they agreed to be accountable for all aspects of the work.
Funding
The authors declare that no funds, grants, or other support were received during the preparation of this manuscript.
Disclosure
The authors report no conflicts of interest in this work.
References
1. Lekhraj Rampal M, Seng LB. Coronavirus disease (COVID-19) pandemic. Med J Malays. 2020;75(2):95.
2. WHO. Coronavirus disease (COVID-19) pandemic. Available from: https://www.who.int/europe/emergencies/situations/covid-19.
3. Marshall JC, Murthy S, Diaz J, et al. A minimal common outcome measure set for COVID-19 clinical research. Lancet Infect Dis. 2020;20(8):e192–e197. doi:10.1016/S1473-3099(20)30483-7
4. Ali AM, Rostam HM, Fatah MH, Noori CM, Ali KM, Tawfeeq HM. Serum troponin, D-dimer, and CRP level in severe coronavirus (COVID-19) patients. Immun Inflamm Dis. 2022;10(3):e582. doi:10.1002/iid3.582
5. Shenoy N, Luchtel R, Gulani P. Considerations for target oxygen saturation in COVID-19 patients: are we under-shooting? BMC med. 2020;18(1):1–6. doi:10.1186/s12916-020-01735-2
6. Huyut MT, Üstündağ H. Prediction of diagnosis and prognosis of COVID-19 disease by blood gas parameters using decision trees machine learning model: a retrospective observational study. Medical Gas Res. 2022;12(2):60. doi:10.4103/2045-9912.326002
7. Mertoglu C, Huyut MT, Arslan Y, Ceylan Y, Coban TA. How do routine laboratory tests change in coronavirus disease 2019? Scandinavian Journal of Clinical and Laboratory Investigation. 2021;81(1):24–33. doi:10.1080/00365513.2020.1855470
8. Tahir Huyut M, Huyut Z, İlkbahar F, Mertoğlu C. What is the impact and efficacy of routine immunological, biochemical and hematological biomarkers as predictors of COVID-19 mortality? Int Immunopharmacol. 2022;105:108542. doi:10.1016/j.intimp.2022.108542
9. Huyut MT, İlkbahar F. The effectiveness of blood routine parameters and some biomarkers as a potential diagnostic tool in the diagnosis and prognosis of Covid-19 disease. Int Immunopharmacol. 2021;98:107838. doi:10.1016/j.intimp.2021.107838
10. Qin C, Zhou L, Hu Z, et al. Dysregulation of immune response in patients with coronavirus 2019 (COVID-19) in Wuhan, China. Clin Infect Dis. 2020;71(15):762–768. doi:10.1093/cid/ciaa248
11. Yang A-P, Liu J-P, Tao W-Q, Li H-M. The diagnostic and predictive role of NLR, d-NLR and PLR in COVID-19 patients. Int Immunopharmacol. 2020;84:106504. doi:10.1016/j.intimp.2020.106504
12. Jin J, Bai P, He W, Wu F, Liu X, Han D. Gender differences in patients with COVID-19: focus on severity and mortality. Front Public Health. 2020;29(8):152. doi:10.3389/fpubh.2020.00152
13. Mertoglu C, Huyut MT, Olmez H, Tosun M, Kantarci M, Coban TA. COVID-19 is more dangerous for older people and its severity is increasing: a case-control study. Med Gas Res. 2022;12(2):51–54. doi:10.4103/2045-9912.325992
14. Hariyanto TI, Putri C, Arisa J, Situmeang RFV, Kurniawan A. Dementia and outcomes from coronavirus disease 2019 (COVID-19) pneumonia: a systematic review and meta-analysis. Arch Gerontol Geriatr. 2021;93:104299. doi:10.1016/j.archger.2020.104299
15. Siahaan YMT, Ketaren RJ, Hartoyo V, Hariyanto TI. Epilepsy and the risk of severe coronavirus disease 2019 outcomes: a systematic review, meta-analysis, and meta-regression. Epilepsy Behav. 2021;125:108437. doi:10.1016/j.yebeh.2021.108437
16. Song P, Li W, Xie J, Hou Y, You C. Cytokine storm induced by SARS-CoV-2. Clin Chim Acta. 2020;509:280–287. doi:10.1016/j.cca.2020.06.017
17. Rahal A, Kumar A, Singh V, et al. Oxidative stress, prooxidants, and antioxidants: the interplay. Biomed Res Int. 2014;2014:761264. doi:10.1155/2014/761264
18. Aykac K, Ozsurekci Y, Yayla BCC, et al. Oxidant and antioxidant balance in patients with COVID-19. Pediatr Pulmonol. 2021;56(9):2803–2810. doi:10.1002/ppul.25549
19. Ayala A, Muñoz MF, Argüelles S. Lipid peroxidation: production, metabolism, and signaling mechanisms of malondialdehyde and 4-hydroxy-2-nonenal. Oxid Med Cell Longev. 2014;2014:360438. doi:10.1155/2014/360438
20. Ahmad R, Hussain A, Ahsan H. Peroxynitrite: cellular pathology and implications in autoimmunity. J Immunoass Immunochem. 2019;40(2):123–138. doi:10.1080/15321819.2019.1583109
21. Alwazeer D, Liu FF-C, Wu XY, LeBaron TW. Combating Oxidative Stress and Inflammation in COVID-19 by Molecular Hydrogen Therapy: mechanisms and Perspectives. Oxid Med Cell Longev. 2021;2021:5513868. doi:10.1155/2021/5513868
22. Miller DD, Li T, Liu RH. Antioxidants and Phytochemicals. Reference Module in Biomedical Sciences. Elsevier; 2014.
23. Drozdz-Afelt JM, Koim-Puchowska BB, Kaminski P. Analysis of oxidative stress indicators in Polish patients with prostate cancer. Environ Sci Pollut Res. 2022;29(3):4632–4640. doi:10.1007/s11356-021-15922-y
24. Traber MG, Stevens JF. Vitamins C and E: beneficial effects from a mechanistic perspective. Free Radic Biol Med. 2011;51(5):1000–1013. doi:10.1016/j.freeradbiomed.2011.05.017
25. Avila-Nava A, Pech-Aguilar AG, Lugo R, Medina-Vera I, Guevara-Cruz M, Gutiérrez-Solis AL. Oxidative Stress Biomarkers and Their Association with Mortality among Patients Infected with SARS-CoV-2 in Mexico. Oxid Med Cell Longev. 2022;2022:1058813. doi:10.1155/2022/1058813
26. Aykac K, Ozsurekci Y, Yayla BCC, et al. Oxidant and antioxidant balance in patients with COVID‐19. Pediatr Pulmonol. 2021;56(9):2803–2810. doi:10.1002/ppul.25549
27. Huyut MT, Huyut Z. Forecasting of Oxidant/Antioxidant levels of COVID-19 patients by using Expert models with biomarkers used in the Diagnosis/Prognosis of COVID-19. Int Immunopharmacol. 2021;100:108127. doi:10.1016/j.intimp.2021.108127
28. Gui-Qiang W, Lei Z, Xia W, Yan-Mei J, Fu-Sheng W. Diagnosis and treatment protocol for COVID-19 patients (tentative 8th edition): interpretation of updated key points. Infect Dis Immun. 2021;1(01):17–19. doi:10.1097/ID9.0000000000000002
29. NHC, NATCM. Diagnosis and Treatment Protocol for COVID-19 Patients (Tentative 8th Edition). Infect Dis Immun. 2020;20(1):1. doi:10.1097/01.ID9.0000733564.21786.b0
30. Lovrić J, Mesić M, Macan M, Koprivanac M, Kelava M, Bradamante V. Measurement of malondialdehyde (MDA) level in rat plasma after simvastatin treatment using two different analytical methods. Period Biol. 2008;110(1):63–68.
31. Griess P. Weselky und Benedikt ueber einege Azoverbindungen. Ber Dtsch Chem Ges. 1879;12:426–428. doi:10.1002/cber.187901201117
32. Sun J, Zhang X, Broderick M, Fein H. Measurement of nitric oxide production in biological systems by using Griess reaction assay. Sensors. 2003;3(8):276–284. doi:10.3390/s30800276
33. Oberley LW, Spitz DR. Assay of superoxide dismutase activity in tumor tissue. Methods Enzymol. 1984;1:457–464.
34. Yenkoyan K, Harutyunyan H, Harutyunyan A. A certain role of SOD/CAT imbalance in pathogenesis of autism spectrum disorders. Free Radic Biol Med. 2018;123:85–95. doi:10.1016/j.freeradbiomed.2018.05.070
35. Asl SH, Nikfarjam S, Majidi Zolbanin N, Nassiri R, Jafari R. Immunopharmacological perspective on zinc in SARS-CoV-2 infection. Int Immunopharmacol. 2021;96:107630. doi:10.1016/j.intimp.2021.107630
36. Huyut MT, Velichko A, Belyaev M. Detection of Risk Predictors of COVID-19 Mortality with Classifier Machine Learning Models Operated with Routine Laboratory Biomarkers. Appl Sci. 2022;12(23):12180. doi:10.3390/app122312180
37. Velichko A, Huyut MT, Belyaev M, Izotov Y, Korzun D. Machine Learning Sensors for Diagnosis of COVID-19 Disease Using Routine Blood Values for Internet of Things Application. Sensors. 2022;22(20):7886. doi:10.3390/s22207886
38. Huyut MT. Automatic Detection of Severely and Mildly Infected COVID-19 Patients with Supervised Machine Learning Models. IRBM. 2022;44:100725. doi:10.1016/j.irbm.2022.05.006
39. Huyut MT, Velichko A. Diagnosis and Prognosis of COVID-19 Disease Using Routine Blood Values and LogNNet Neural Network. Sensors. 2022;22(13):4820. doi:10.3390/s22134820
40. Hariyanto TI, Japar KV, Kwenandar F, et al. Inflammatory and hematologic markers as predictors of severe outcomes in COVID-19 infection: a systematic review and meta-analysis. Am J Emerg Med. 2021;41:110–119. doi:10.1016/j.ajem.2020.12.076
41. Tarteret P, Strazzulla A, Rouyer M, et al. Clinical features and medical care factors associated with mortality in French nursing homes during the COVID-19 outbreak. Int J Infect Dis. 2021;104:125–131. doi:10.1016/j.ijid.2020.12.004
42. Lippi G, Plebani M. Laboratory abnormalities in patients with COVID-2019 infection. Clin Chem Lab Med. 2020;58(7):1131–1134. doi:10.1515/cclm-2020-0198
43. He F, Deng Y, Li W. Coronavirus disease 2019: what we know? J Med Virol. 2020;92(7):719–725. doi:10.1002/jmv.25766
44. Yang M, Hon K-LE, Li K, Fok T-F, Li C-K. The effect of SARS coronavirus on blood system: its clinical findings and the pathophysiologic hypothesis. J of Exp Hematol. 2003;11(3):217–221.
45. Lippi G, Plebani M, Henry BM. Thrombocytopenia is associated with severe coronavirus disease 2019 (COVID-19) infections: a meta-analysis. Clin Chim Acta. 2020;506:145–148. doi:10.1016/j.cca.2020.03.022
46. Fathi N, Rezaei N. Lymphopenia in COVID-19: therapeutic opportunities. Cell Biol Int. 2020;44(9):1792–1797. doi:10.1002/cbin.11403
47. Vafadar Moradi E, Teimouri A, Rezaee R, et al. Increased age, neutrophil-to-lymphocyte ratio (NLR) and white blood cells count are associated with higher COVID-19 mortality. Am J Emerg Med. 2021;40:11–14. doi:10.1016/j.ajem.2020.12.003
48. Zahorec R. Neutrophil-to-lymphocyte ratio, past, present and future perspectives. Bratisl Lek Listy. 2021;122(7):474–488. doi:10.4149/BLL_2021_078
49. Farid E, Sridharan K, Alsegai OAM, et al. Utility of inflammatory biomarkers in patients with COVID-19 infections: Bahrain experience. Biomark Med. 2021;15(8):541–549. doi:10.2217/bmm-2020-0422
50. Karagol C, Tehci AK, Gungor A, et al. Delta neutrophil index and C-reactive protein: a potential diagnostic marker of multisystem inflammatory syndrome in children (MIS-C) with COVID-19. Eur J Pediatr. 2022;181(2):775–781. doi:10.1007/s00431-021-04281-y
51. Soni M, Gopalakrishnan R, Vaishya R, Prabu P. D-dimer level is a useful predictor for mortality in patients with COVID-19: analysis of 483 cases. Diabetes Metab Syndr. 2020;14(6):2245–2249. doi:10.1016/j.dsx.2020.11.007
52. Shakaroun DA, Lazar MH, Horowitz JC, Jennings JH. Serum Ferritin as a Predictor of Outcomes in Hospitalized Patients with Covid-19 Pneumonia. J Intensive Care Med. 2022;8850666221113252. doi:10.1177/08850666221113252
53. Mehri F, Rahbar AH, Ghane ET, Souri B, Esfahani M. Changes in oxidative markers in COVID-19 patients. Arch Med Res. 2021;52(8):843–849. doi:10.1016/j.arcmed.2021.06.004
54. Dominic P, Ahmad J, Bhandari R, et al. Decreased availability of nitric oxide and hydrogen sulfide is a hallmark of COVID-19. Redox Biol. 2021;43:101982. doi:10.1016/j.redox.2021.101982
55. Fang W, Jiang J, Su L, et al. The role of NO in COVID-19 and potential therapeutic strategies. Free Radic Biol Med. 2021;163:153–162. doi:10.1016/j.freeradbiomed.2020.12.008
56. Haagmans BL, Osterhaus AD. Coronaviruses and their therapy. Antiviral Res. 2006;71(2–3):397–403. doi:10.1016/j.antiviral.2006.05.019
57. Star RA. Nitric Oxide. Am J Med Sci. 1993;306(5):348–358. doi:10.1097/00000441-199311000-00015
58. Sandstead HH, Prasad AS, Penland JG, et al. Zinc deficiency in Mexican American children: influence of zinc and other micronutrients on T cells, cytokines, and antiinflammatory plasma proteins. Am J Clin Nutr. 2008;88(4):1067–1073. doi:10.1093/ajcn/88.4.1067
59. Trumbull KA, Beckman JS. A role for copper in the toxicity of zinc-deficient superoxide dismutase to motor neurons in amyotrophic lateral sclerosis. Antioxid Redox Signal. 2009;11(7):1627–1639. doi:10.1089/ars.2009.2574
60. Muhammad Y, Kani YA, Iliya S, et al. Deficiency of antioxidants and increased oxidative stress in COVID-19 patients: a cross-sectional comparative study in Jigawa, Northwestern Nigeria. SAGE Open Medicine. 2021;9:2050312121991246. doi:10.1177/2050312121991246
61. Estevez AG, Crow JP, Sampson JB, et al. Induction of Nitric Oxide -- Dependent Apoptosis in Motor Neurons by Zinc-Deficient Superoxide Dismutase. Science. 1999;286(5449):2498–2500. doi:10.1126/science.286.5449.2498
62. Crow JP, Ye YZ, Strong M, Kirk M, Barnes S, Beckman JS. Superoxide Dismutase Catalyzes Nitration of Tyrosines by Peroxynitrite in the Rod and Head Domains of Neurofilament-L. J Neurochem. 2002;69(5):1945–1953. doi:10.1046/j.1471-4159.1997.69051945.x
63. Yaghoubi N, Youssefi M, Jabbari Azad F, Farzad F, Yavari Z, Zahedi Avval F. Total antioxidant capacity as a marker of severity of COVID-19 infection: possible prognostic and therapeutic clinical application. J Med Virol. 2022;94(4):1558–1565. doi:10.1002/jmv.27500
64. Chiscano-Camón L, Ruiz-Rodriguez JC, Ruiz-Sanmartin A, Roca O, Ferrer R. Vitamin C levels in patients with SARS-CoV-2-associated acute respiratory distress syndrome. Crit Care. 2020;24(1):522. doi:10.1186/s13054-020-03249-y
65. Sinnberg T, Lichtensteiger C, Hill-Mündel K, et al. Vitamin C Deficiency in Blood Samples of COVID-19 Patients. Antioxidants. 2022;11(8):1580. doi:10.3390/antiox11081580
66. Al-Rashedi NAM, Alburkat H, Hadi AO, et al. High prevalence of an alpha variant lineage with a premature stop codon in ORF7a in Iraq, winter 2020–2021. PLoS One. 2022;17(5):e0267295. doi:10.1371/journal.pone.0267295
67. GISAID. Latest data from China resembles known circulating variants 17-Jan-2023. Available from: https://gisaid.org/.
© 2023 The Author(s). This work is published and licensed by Dove Medical Press Limited. The full terms of this license are available at https://www.dovepress.com/terms.php and incorporate the Creative Commons Attribution - Non Commercial (unported, v3.0) License.
By accessing the work you hereby accept the Terms. Non-commercial uses of the work are permitted without any further permission from Dove Medical Press Limited, provided the work is properly attributed. For permission for commercial use of this work, please see paragraphs 4.2 and 5 of our Terms.